Data Architecture
Data Architecture
Create A Data Architecture That Maximizes Your Business Value.
Data architecture, enriched by AI, is a discipline that outlines an organization’s data assets, charts the flow of data across systems, and presents a data management strategy. It harnesses AI to automate tasks, gain insights, enhance real-time data processing, and ensure data security, ultimately ensuring that data is maintained accurately and businesses’ information needs are met effectively.
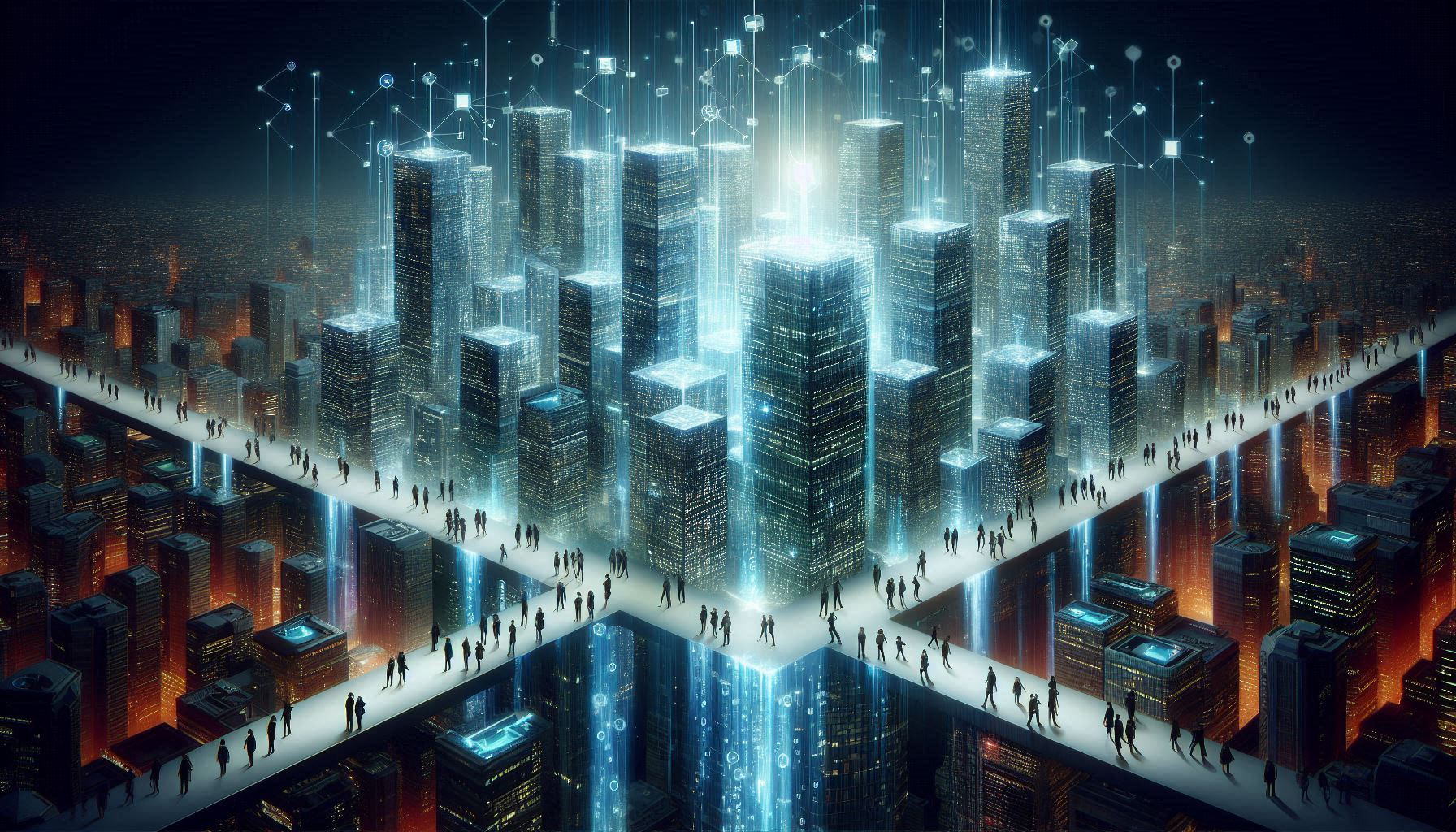
Why Is Data Architecture Important?
A data strategy aligned with organizational objectives and priorities relies on a robust data architecture. This architecture, combined with data engineering and data preparation, plays a crucial role in improving data integration and quality. AI technologies enhance this process by automating data tasks, extracting valuable insights, and ensuring real-time data processing. Furthermore, AI enables efficient data governance and the establishment of internal data standards, contributing to a comprehensive data management framework that supports organizational goals.
Benefits of Data Architecture
Incorporating AI into data architecture accelerates data quality improvements, streamlines data integration processes, and reduces data storage costs. It achieves this by adopting an enterprise-wide perspective, moving beyond domain-specific data modelling and database design. AI’s capabilities for data analysis and pattern recognition make data architecture even more powerful in harnessing valuable insights for a wide range of applications.
Key Steps For Creating A Data Architecture
To create a data architecture, data management teams must collaborate closely with business leaders and other end users. If they do not, it may be out of sync with corporate plans and data requirements. Engaging with top executives to get their support and meeting with users to understand their data requirements are both important.
Other steps include:
- Data governance directives are used to assess data threats.
- Data flows, as well as data lifecycle and data lineage information, may be tracked.
- Describe and evaluate the current data management technology infrastructure.
- Make a plan for the data architecture deployment initiatives.